Executive summary
Despite its many positives, rapid advances in AI are having an escalating impact on the environment, particularly due to massive energy and resource consumption. This is being seen across the AI pipeline, from the manufacture of hardware through the training and use of AI models and on to the data centers that support the delivery of these services. The AI industry is also a growing source of e-waste, and so players are pivoting to a much greater focus on sustainability. This includes using more environmentally friendly hardware, developing and training AI models that are much less energy-intensive, and reducing the carbon footprint of data centers by improving energy efficiency and switching to renewable energy sources. The move to improve the sustainability of AI has thus emerged alongside growing investment in renewable-powered green data centers, and so against this backdrop, if Thailand is to seize the opportunities opening up as the tech frontier moves forward, it is imperative that the share of energy coming from renewables is increased and that the nation’s clean energy ecosystem is improved. Success in this will then help the country attract growing investment inflows from global leaders in AI.
Will progress in AI worsen climate change?
Artificial intelligence, or AI, has made stunning advances in recent years, and around the world, a broad range of related technologies is finding widespread adoption in both business and day-to-day life. However, much of society remains unaware of the cost that is paid for this progress. Indeed, there is a widespread assumption that digital technologies have only a limited environmental impact and are not significant sources of greenhouse gases compared to more traditional industries like energy and transport. In reality, the situation is very different, and as a result of its enormous energy consumption, the tech industry is a major contributor to indirect carbon emissions. Thus, the global information and communications technology industry is responsible for 4% of worldwide carbon emissions1/, against 3% for the much more commonly vilified air transport industry.
Within the tech sector, AI’s environmental impacts are a growing source of concern, and the surge in demand for these services is driving a vast increase in consumption of both physical resources and energy. In particular, the data centers that provide much of the industry’s physical infrastructure are a huge drain on local energy supplies, both for the servers themselves and for their cooling systems, which then require additional sources of water, and as a result, AI’s carbon and water footprints are enormous. A further consequence of the breakneck pace of technological development within the AI industry is that hardware rapidly becomes out-of-date and as this is replaced and its value crashes, the global supply of e-waste expands yet further. Details of the industries sizeable impacts are given below.
AI’s energy consumption and associated carbon emissions
Developing generative AI (or GenAI) systems based on large language models (LLMs) is extremely energy intensive, and this is then a significant source of carbon. For example, GPT-3 (Generative Pre-trained Transformer 3, or the model that initially powered ChatGPT) was trained on a database containing 500 billion words of text. This required around 1,300 MWh of power, equivalent to the power consumed by more than 120 average US homes in a year. The training generated 500 tonnes of carbon emissions, comparable to 500 flights from New York to San Francisco or the lifetime emissions of 8 cars, including fuel (Figure 1). GPT-3 was superseded by GPT-4, which has many more parameters2/, and this is estimated to have increased energy consumption 50-fold3/.
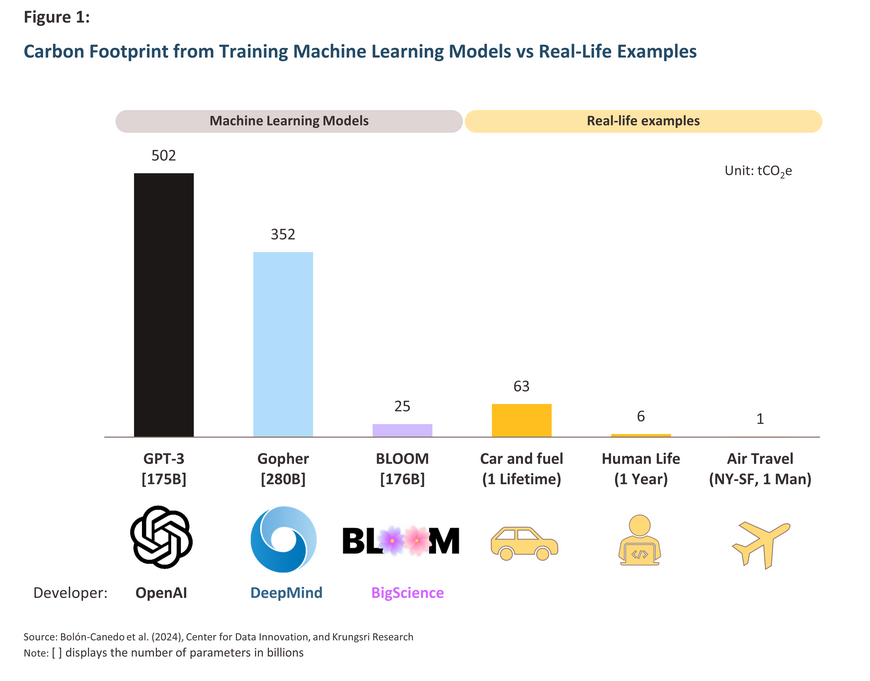
However, a large body of research shows that because generating responses requires a hugely complex model to be run in real-time, the major source of energy use is in AI ‘inference’4/. Thus, a single query on ChatGPT uses as much energy as running a 5W LED light for 1 hour and 20 minutes5/, although the exact energy-intensity of the request will vary depending on: (i) the type of response being generated, with image generation consuming around 1,000-times as much energy as a much simpler text classification task (Figure 2); and (ii) the type of model being used, since energy use increases with the number of parameters in the model6/.
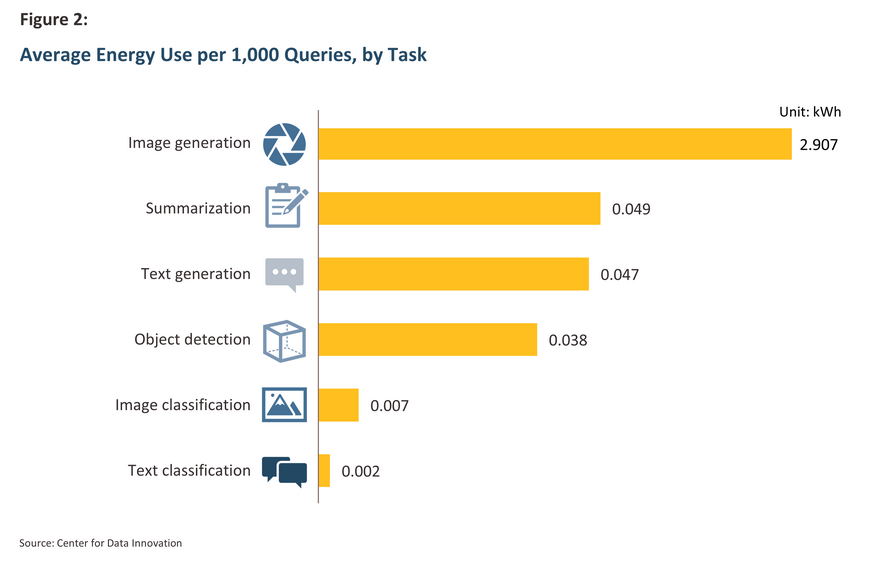
The AI industry’s carbon footprint is largely a result of the operation of data centers since this is where the enormous quantities of data needed for training and running AI models is gathered, stored and deployed. Data centers are thus a particularly energy-intensive part of the AI infrastructure, using 10-50 times as much energy as general offices7/. Around 40%-50% of this energy is used to power hardware, with another 30%-40% going to the cooling equipment needed to dissipate the heat that all this machinery generates8/.
Given the explosive growth in AI, the International Energy Agency (IEA)9/ estimates that by 2026, global consumption of electricity by data centers will double from its 2022 level to around 1,000 TWh, approximately equal to the entire demand generated by Japan. The IEA thus sees data centers accounting for a third of the increase in demand for electricity in the US over 2025-2026, and the central role played by data centers in driving increases in electricity consumption will be repeated in the EU and China (Figure 3).
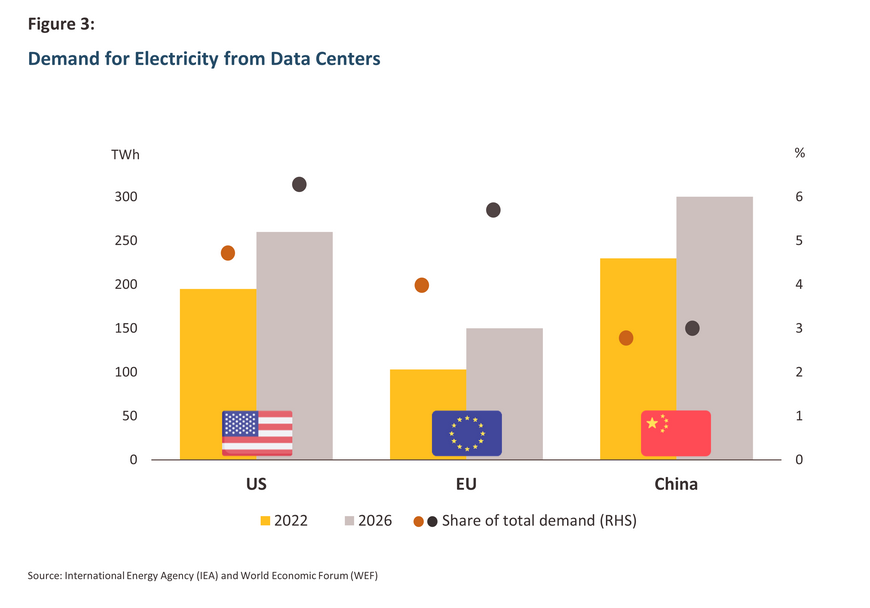
Water consumption
In addition to placing a major strain on electricity supplies, data centers also require significant inputs of fresh water. This is needed because the servers that are at the heart of AI operations generate a considerable amount of heat, and to prevent this damaging hardware, this needs to be dissipated. Thus, training the GPT-3 model in a US data center is estimated to have consumed 5.4 million liters of water, while every 10-50 ChatGPT (GPT-3) queries require the equivalent of a further 0.5 liters of drinking water. Naturally, the increased complexity of GPT-4 means that its cooling needs will be higher, adding to the drain on water resources10/.
Beyond these immediate needs, the AI infrastructure is built on advanced hardware (primarily servers and data storage units) all of which make extensive use of chips, and tracking further back in the supply chain, access to water is a crucially important factor in facilitating chip production. This is because water is used throughout fabs for cooling machinery and cleaning wafers, and so a 2024 report by S&P Global indicates that global chip manufacture uses as much water as all of Hong Kong, while an average fab consumes 10 million gallons of purified water (approximately 38 million liters) per day, equivalent to the average daily consumption of 33,000 US households11/. Moreover, chip production also requires metals such as copper, aluminium and lithium, and the mining processes that yield these ores is also an important source of water contamination and other unwanted environmental impacts.
E-waste
The rapid pace of development in AI means that there is a constant cycle of upgrades to the hardware used in data centers, and on average, this equipment has a lifespan of just 2-5 years. At the end of this time, this will usually be disposed of, but hardware will typically contain heavy metals such as lead, mercury and cadmium. These are toxic and so if improper disposal of e-waste results in contamination of land or water sources, this can pose a threat to both humans and the broader ecosystem.
Research published in Nature Computational Science at the end of October 2024 indicates that the GenAI industry will generate an additional 1.2-5 million tonnes of e-waste over 2023-2030, and although this represents only a relatively small fraction of the 62 million tonnes of e-waste created globally in 2022, this is growing rapidly. Thus, between 2023 and 2030, it is possible that annual GenAI e-waste will increase almost 1000-fold, or to a level that is equal to the quantity of waste generated in the production of more than 10 billion iPhone 15 Pros12/.
It is clear from the above that the emergence and rapid development of AI brings both gains and losses, and in the case of the latter, this includes the additional demand placed on supplies of energy and water, together with a broad range of negative environmental impacts. In light of this, the drive towards sustainable AI, that is, a new model for AI that continues to generate the maximum benefits but that also minimizes negative consequences for both the environment and society, is taking a much more central place in public discourse.
What is sustainable AI and why does it matter?
Sustainable AI aims to develop the AI industry in a way that emphasizes the importance of sustainability across the AI lifecycle in areas as diverse as sourcing inputs and hardware, developing data centers and the rest of the industry’s infrastructure, gathering and storing data, training and testing models, deploying and using these, and managing e-waste at the end of the lifecycle (Figure 4). Current trends in sustainable AI are centered around reducing the environmental impacts connected with the industry’s enormous energy and resource consumption, which is in keeping with global efforts to reach net-zero emissions across the international economy. Given this, sustainable AI may also be referred to as ‘eco-friendly AI’ and ‘green AI’, but there is no significant difference between the meaning of these terms.
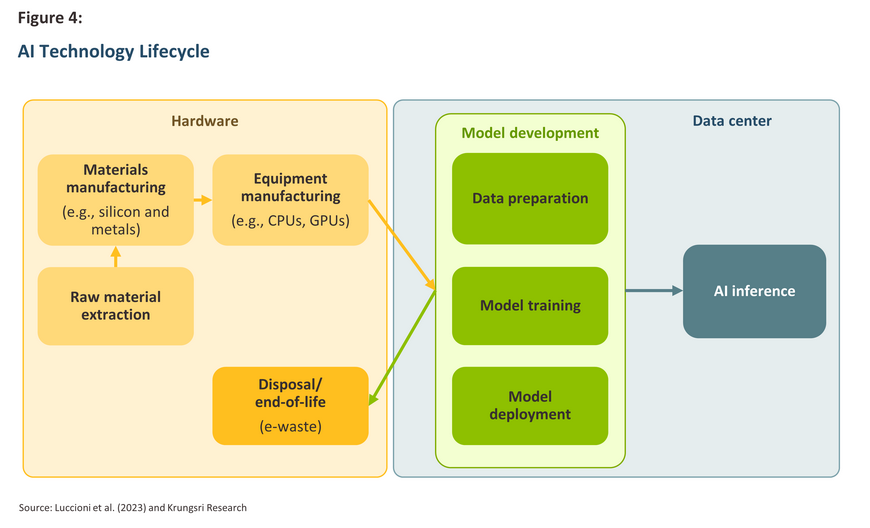
Efforts to improve the sustainability of the AI industry follow three main tracks: (i) hardware optimization; (ii) model optimization; and (iii) data center optimization. Details of these three paths are given below.
Hardware optimization: Using hardware efficiently and making this more environmentally friendly
The AI lifecycle begins at the most upstream position of the supply chain, where ores and minerals are mined for use in the manufacture of the electronics parts and components contained in AI hardware, such as central processing units (CPUs)13/ and graphics processing units (GPUs)14/.
Environmental impacts begin at this early stage of the supply chain. It is estimated that production of the GPUs used to train the BLOOM model, an LLM developed by BigScience15/, accounted for 22% of all carbon emissions associated with its training stage. Sustainable AI thus encourages the use of environmentally friendly inputs in the hardware production process, for example by sourcing recycled steel and aluminium, bioplastics, and rare earth alternatives. In addition, it is important to focus on optimizing the use of components and equipment, in particular by maximizing their lifespan since this will help to reduce both initial demand and the quantity of e-waste that has to be processed.
AI-specific hardware is also being designed that improves on the efficiencies achieved by current technologies, thus lowering energy consumption. One prominent example of this is tensor processing units (TPUs), a kind of application-specific integrated circuit (ASIC) developed by Google. When completing the particular mathematical operations necessary for deep learning, TPUs are faster, more accurate, and more efficient (in terms of energy consumption) than the more general-purpose CPUs and GPUs currently in use16/.
Model optimization: Ensuring that AI models are less energy-hungry
The extent of the carbon emissions associated with designing, training and using an AI model will be directly impacted by the latter’s size and complexity, with larger models that are built on enormous datasets requiring more extensive and higher performing hardware, which together with the associated cooling mechanisms will add to energy demands. As such, one approach to cutting AI’s carbon footprint is to use various algorithmic optimization techniques to design more efficient and less energy-intense AI models. At present, these efforts are focused on cutting models’ memory footprints and computational complexity, in particular by: (i) post-training pruning of artificial neural networks (ANNs), which can cut the model’s size without impacting its abilities; (ii) quantization, which reduces the precision of the model’s internal weights, often by converting these from floating point to integer representations, thereby helping to improve the memory load without radically impacting accuracy; (iii) distillation, or the transfer of knowledge from a large and complex ‘teacher model’ to a smaller and simpler ‘student model’, which should be able to achieve similar levels of performance as the teacher; (iv) using ‘flash attention’ to better allocate memory and reduce computational demands during both the training and inference stages of LLM usage17/.
A further development that goes by the name of ‘tiny machine learning’ (TinyML) is also attracting attention since by making use of both pruning and quantization18/, AI products can be deployed on devices with only limited memory and power.
Data center optimization: Improving efficiency and increasing the use of renewables
The high-performance servers, data storage units, and extensive cooling systems that fill data centers are all significant consumers of electricity, whether that is when training and using AI models (i.e., dynamic energy consumption) or when powered up but unused (i.e., idle energy consumption). It is therefore possible to reduce data centers’ carbon footprint by increasing their energy efficiency, which can be achieved by using free or passive cooling technologies that take advantage of naturally occurring heat differentials to shift heat out of the data center, server virtualization, which can help reduce the number of physical servers that are required, and installing data center infrastructure management systems (DCIM) to better track electricity demand. In terms of monitoring the effectiveness of these strategies, the primary tool involves measuring power usage effectiveness (PUE)19/, with the hope that this is driven as close as possible to 1.
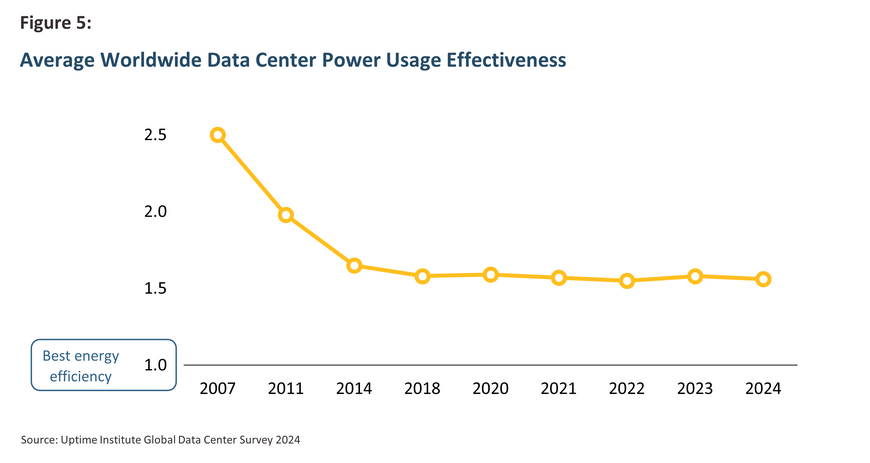
Nevertheless, although the overall efficiency of data centers has been improving globally, average PUE values did not change dramatically over 2020-2024 (Figure 5). Fortunately, another approach to reducing the industry’s carbon footprint that can be deployed simultaneously is switching to renewable energy sources like wind and solar by situating data centers in regions with ready access to clean energy. Data from Cloudscence as of October 202420/ shows that the energy supply in countries in North America and Europe, which are home to a disproportionately high number of data centers, is less carbon-intensive than in countries in the Asia-Pacific region, where electricity production is still more reliant on the use of fossil fuels (Figure 6).
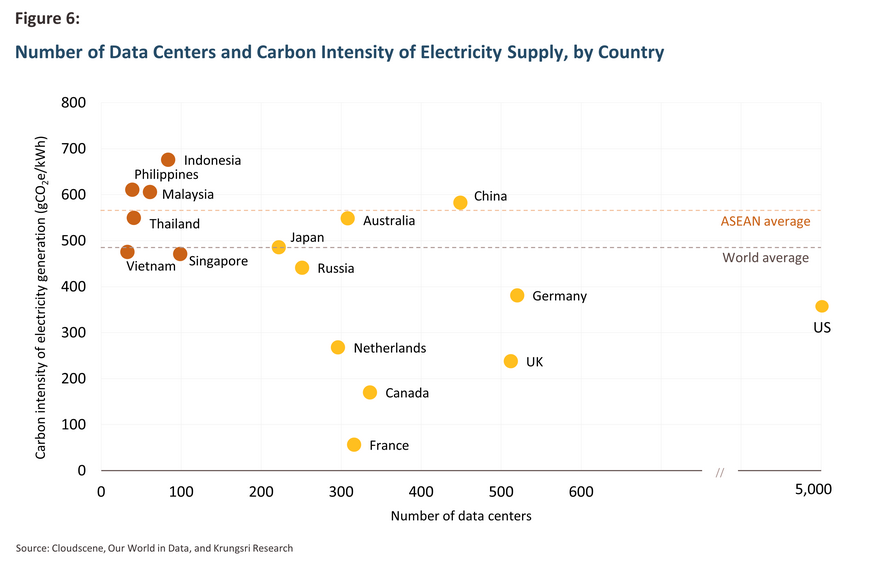
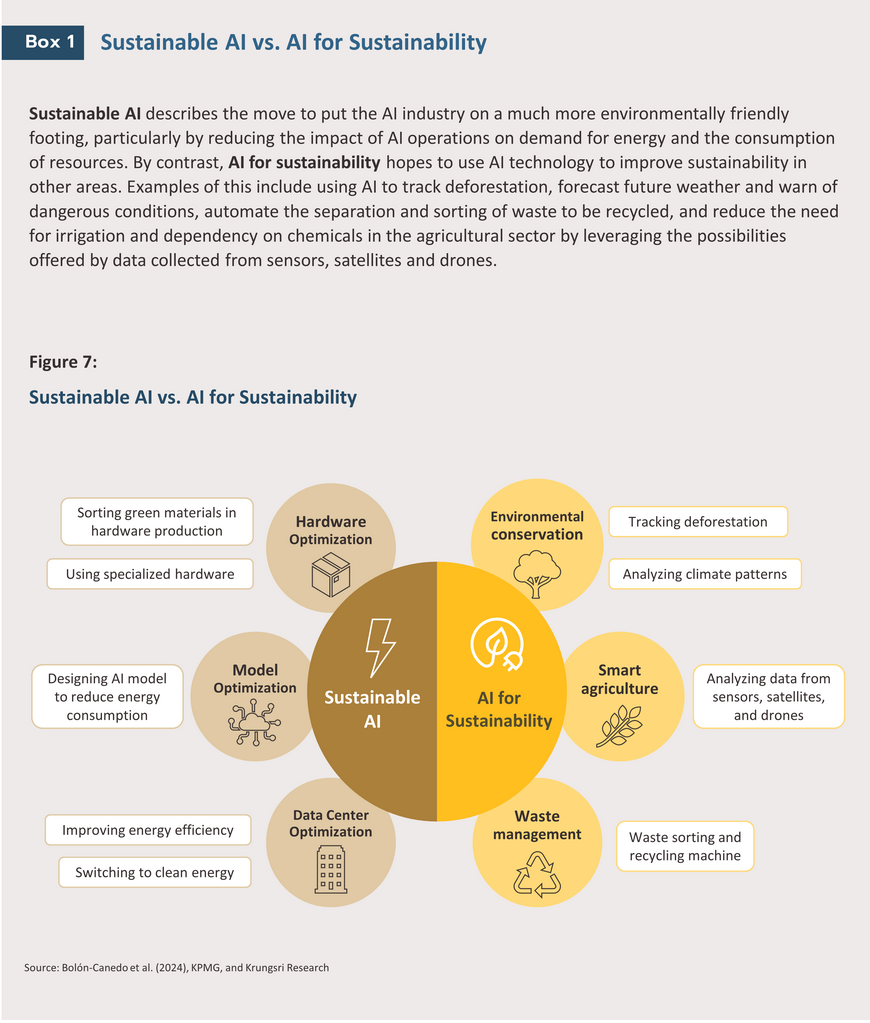
Global efforts to green the AI industry
Global tech giants, which double up as leaders in the field of AI, have all announced sustainability goals, and so these companies are now attempting to increase the sustainability of their AI operations. Some interesting examples of how this is being realized are described below (Figure 8).
Google is attempting to cut its energy consumption and so reduce its carbon footprint through four channels21/: (i) designing hardware that is optimized specifically for deep learning contexts (e.g., TPUs and neuromorphic chips) that can be as much as 2-5-times more efficient; (ii) using more efficient models, such as ‘sparse’ models that ignore zero-value weights and so are smaller and less memory-intense; (iii) moving from the use of traditional data centers to cloud-based data centers22/, which can cut emissions by 25%-50%; and (iv) investing in sources of renewable power for use in data centers. Google aims to be entirely supplied by clean energy by 2030.
Microsoft is working on developing more energy-efficient models, including scaled down 1-bit LLMs (e.g., BitNet b1.58) that are able to replicate the performance of much more accurate 16-bit models but because these are less computationally complex, they are much less energy-intensive23/. Microsoft is also constructing green data centers in Sweden, where renewables are abundant24/, and underwater (Microsoft’s project Natick25/), since this allows for passive cooling using seawater. Beyond this, the company is improving data center efficiency by dynamically diverting surplus energy across server infrastructure26/. Microsoft has set the ambitious goal of becoming carbon negative by 2030 (i.e., the company hopes to draw down more carbon than it emits).
Amazon was the global leader in corporate investment in wind and solar power for four consecutive years, from 2020 to 2023. In 2023, the company was able to report that over 90% of its energy consumption (including supply to data centers) came from renewables27/. Amazon hopes to be 100% renewables-based by 2025.
Meta’s sustainability efforts have focused on its data centers’ use of water resources, and so the company has set a target of being ‘water positive’ (i.e., of restoring water resources to a level that more than offsets corporate water consumption) by 203028/. Meta data centers are also all certified to international standards for energy efficiency.
In addition to these companies, Nvidia (the market leading manufacturer of GPUs) is working on new energy-saving chips for use in large models. These include the Grace Hopper Superchip, which can cut energy consumption by up to 10 times29/, and the Blackwell GPU, which is up to 20-times more efficient than standard CPUs. Nvidia is also a major consumer of renewable energy, and in the 2024 financial year, 76% of its electricity supply came from renewable sources, thereby helping to keep the company on track to meet its 2025 goal of being powered entirely by clean energy30/. Likewise, OpenAI is also focused on improving the energy demands entailed in designing and training GenAI models, and so it has partnered with Microsoft to deploy its services within renewables-powered data centers31/.
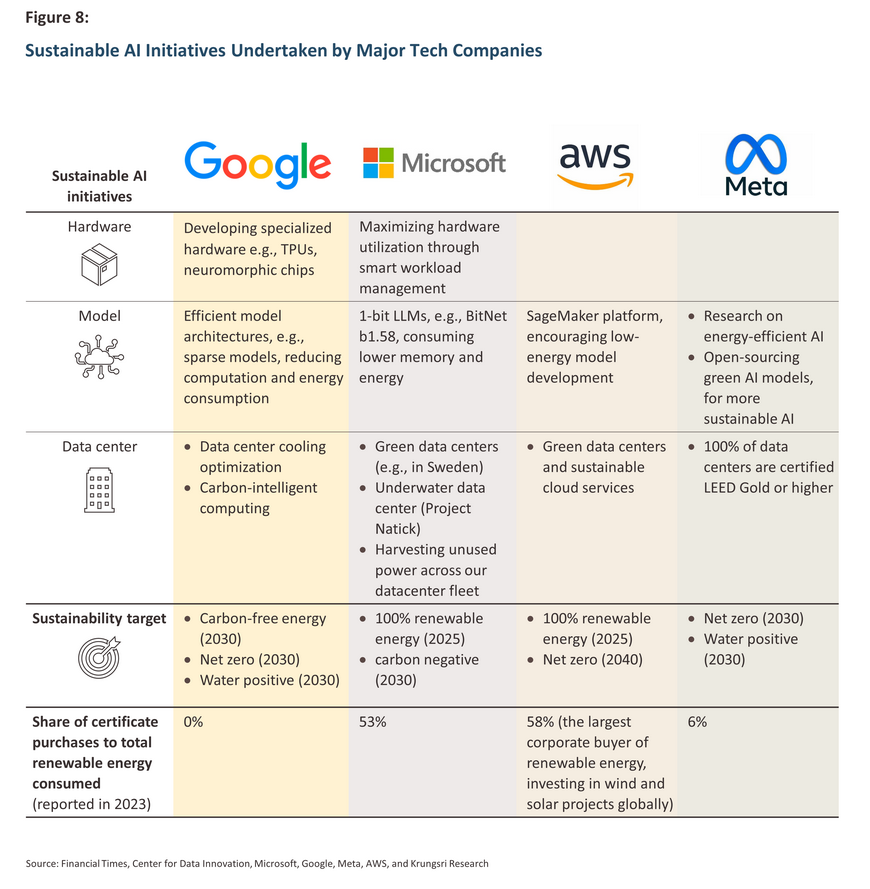
It is clear from the above that major corporations active in the AI industry are all following a similar path. These have thus set ambitious targets for achieving net zero between 2030 and 2040, significantly ahead of the 2050 that most countries and industries are targeting. As part of this, many of these companies are also trying to ensure that by 2025, 100% of their electricity demand is met by supply from renewables, and to this end, a range of financial and market mechanisms are being deployed, including renewable energy certificates (RECs) and carbon credits. Nevertheless, progress towards sustainable AI remains limited, and much work is still at the stage of research and development. Moving forward will therefore require an additional push from changes to the regulatory environment, and so this will be discussed next.
Current sustainable AI regulatory environment
Despite the rapid global rise in worries over sustainability, regulations governing AI and the environment are still only at their initial stage of development. Nevertheless, the EU has been a pioneer in this area, and the EU AI Act32/, which came into effect on August 1, 2024, is the first such legislation in the world33/. This bears on the development of AI and requires that AI companies take into account issues related to energy efficiency, resource use, human rights, and discrimination. The AI Act also forces companies active in this space to adopt a risk-based approach34/, and so developers of general-purpose AI models (GPAI), such as ChatGPT, are required to report data relating to energy use and to assess energy efficiency and consumption use across the AI lifecycle. However, the EU AI Act is focused more on protecting basic human rights and securing livelihoods than on addressing environmental issues.
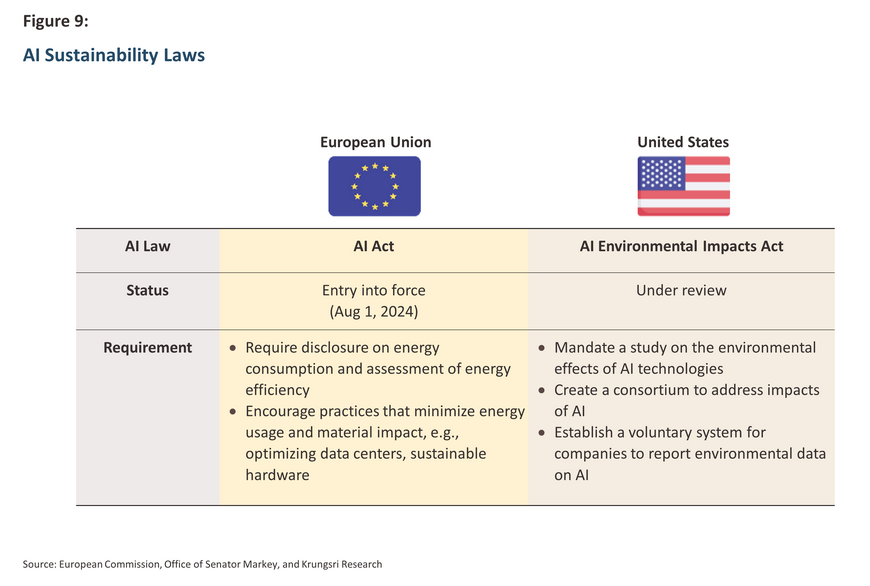
On the other side of the Atlantic, although the US is the world leader in AI, it still lacks explicit AI legislation, but in February 2024, a group of senators and representatives35/ presented a draft of the proposed Artificial Intelligence Environmental Impacts Act. This proposes that the Environmental Protection Agency research the environmental impacts across the length of the AI lifecycle, including areas such as energy use, carbon emissions, and management of e-waste. The draft act also calls for the establishment of the AI Environmental Impacts Consortium and the development of a system for the voluntary reporting of environmental impacts arising from the AI industry. In Canada, the Pan-Canadian Artificial Intelligence Strategy has been in place since 2017. This aims to develop an AI ecosystem that is focused on generating benefits for society and the environment, that is, it is a version of AI for Sustainability. However, the draft Artificial Intelligence and Data Act, which is similar to the EU AI Act, lacks a strong emphasis on the environmental side of the AI industry36/.
Turning to Asia, the authorities in China are in the process of drafting new AI regulations but as yet, there are no clear controls directing the development of sustainable AI. By contrast, although there is no legislation on sustainable AI in Singapore, officials have established the Model AI Governance Framework to cover the ethical and responsible use of AI. The Singaporean authorities have also set up the ‘Green Data Centre Roadmap’, which aims to improve energy efficiency within data centers and in particular, to reduce the average PUE to no more than 1.3 by 203037/. In summary, while current regulations that bear on AI sustainability remain relatively lax, these are expected to evolve and to tighten as the AI industry matures and the global consensus on the importance of environmental issues firms up.
Krungsri Research view: The future of sustainable AI and what it means for Thailand
Future directions in AI
On the one hand, AI will have an important role to play in supporting the global shift to sustainability, but on the other, the development and use of AI places a heavy burden on resource use and energy demand, and so AI will impact sustainability on both sides of the equation. In light of these conflicting effects, it is important that further development of the AI industry gives due weight to environmental considerations, thereby allowing society to draw the maximum benefits from these technologies while incurring a minimum of environmental costs. The key trends in sustainable AI development are as follows.
-
Efforts to give the AI industry a more environmentally friendly structure across supply chains and lifecycles will include the following. (i) Using specialized hardware and in particular chips will allow for highly complex calculations to be carried out but with lower memory and power demands than at present. Developing equipment that can harvest energy from surrounding light, movement and heat will also help hardware operate independently of external sources of electricity. (ii) Better design of AI models and algorithms will help to conserve energy. This will include better sizing models to their use case and taking advantage of edge computing38/ to carry out computational tasks close to sources of data and end users, thus reducing the need for energy to power the transmission of data over potentially long distances to and from data centers. (iii) Data centers’ carbon footprint will be reduced through investment in sites that are powered by renewable energy, improving the efficiency of cooling systems, using AI to better manage power supplies, and moving to cloud-based data centers. Although at present, many of these issues are still at the stage of research and development, these will increasingly be deployed commercially as the impacts of climate change intensify.
-
Explainable AI (XAI) refers to AI models that are able to explain to users their reasoning and the stages that they went through to produce a response39/, which then allows developers to better analyze how energy is being used during inferencing and ultimately, this should improve a model’s overall efficiency. For example, if an XAI model reports that preparing data for a particular stage of the reasoning process uses too much energy, developers may be able to revise or sidestep these processes. In addition, XAI will help companies better track their own carbon emissions and to improve planning for how this can be reduced.
-
A greater regulatory focus on AI sustainability will extend this to encompass areas similar to those covered by the EU regulations, which require AI developers to report on their energy usage and encourage greater energy efficiency. Regulations may also mirror Singapore’s support for green data centers. Globally, as consumer awareness of AI’s environmental impacts becomes more widespread, coverage of issues relating to AI sustainability in regulatory frameworks is expected to become both stricter and more common. Moreover, because global tech giants, which are also key players in the AI industry, have set such challenging environmental targets, there will be rising pressure on countries that are connected to AI value chains to push through changes to policies that encourage increased attention to AI sustainability.
Opportunities for Thailand and how best to prepare for these
With the tech industry tilting to the development of sustainable AI and new opportunities opening up in the wake of this, it is imperative that Thailand moves rapidly to take advantage of these changes and in particular, capture a share of rising global investment in green data centers. Thailand is well placed to profit from these developments given its position in the heart of Southeast Asia, the advanced state of its digital infrastructure, the country’s high level of energy security (reflected in Thailand’s low ‘loss of load expectation’, or LOLE), high levels of internet penetration and uptake of digital services, and the government’s investment promotion policies40/. As such, investment inflows into Thailand-based data centers have been significant, and as of November 2024, 47 applications connected to data centers and cloud computing services (valued at almost THB 200 billion) had been submitted to the Board of Investment for investment support41/. Global tech leaders including Amazon Web Services (AWS)42/, Microsoft43/ and Google44/ have also all announced plans to invest in Thailand, but because these companies have set aggressive net zero goals, it is important that they have access to sources of clean energy, and this is another reason why it is important that Thailand makes greater efforts to accelerate its domestic energy transition. This will include the following.
-
Thailand needs to increase the share of renewables in the national energy mix. At present, there are 41 data centers in operation in Thailand, placing the country 4th in the ASEAN region after Singapore, Indonesia, and Malaysia, but the country’s energy supply remains more carbon intensive than either Singapore’s or Vietnam’s, and this may influence future investment decisions45/. To clear these potential blockages to increased FDI inflows, officials should accelerate the implementation of the PDP 2024 (Thailand Power Development Plan 2024-2037), which aims to increase the share of energy coming from renewables to 51% of the total by 203746/, a sharp upgrade on the earlier target of 36% set in the 2021-2030 plan. If PDP 2024 is successfully implemented, the largest single source of renewables will be solar, which would account for 16% of all power supply, but reaching these goals will require the use of incentives and an easing of the blockages that prevent small-scale generators selling solar-powered electricity.
-
Improving access to sources of clean energy should also be a top priority. At present, it can be difficult for companies to access supplies of clean energy, and so those looking to set up green data centers may have to locate these on an industrial estate or install their own solar rooftop and solar farm nearby47/. However, the government is attempting to liberalize the supply of electricity by allowing suppliers and companies to agree ‘direct power purchasing agreements’ (direct PPAs), which in turn depend on third party access (TPA) to the electricity infrastructure and the national grid. These changes will then help data centers gain access to power supplied by private-sector generators. Moreover, the development of the ‘utility green tariff’ (UGT) and ‘renewable energy certificates’ (RECs) will further allow companies to validate their use of renewables, thus helping these achieve their net zero goals48/.
Ultimately, the convergence of two global megatrends, namely explosive growth in AI and rising awareness of the central importance of sustainability, will help to drive the development of a new form of sustainable AI that pays much closer attention to the industry’s environmental impacts. Thanks to its position within global tech value chains, Thailand is well positioned to reap enormous benefits from these trends, but doing so will require that the country develops its clean energy infrastructure and supporting ecosystem. Success in this will then allow Thailand to gain a march on its competitors and to attract what will surely be growing investment inflows from the global tech giants.
References
Bolón-Canedo, Verónica et al. (2024). “A review of green artificial intelligence: Towards a more sustainable future” Retrieved from https://www.sciencedirect.com/science/article/pii/S0925231224008671#fn20
Castro, Daniel. (2024). “Rethinking Concerns About AI’s Energy Use”. Retrieved from https://www2.datainnovation.org/2024-ai-energy-use.pdf
International Energy Agency. (2024). “Electricity 2024: Analysis and forecast to 2026.” Retrieved from https://iea.blob.core.windows.net/assets/18f3ed24-4b26-4c83-a3d2-8a1be51c8cc8/Electricity2024-Analysisandforecastto2026.pdf
Li, Pengfei et al. (2023). "Making ai less" thirsty: Uncovering and addressing the secret water footprint of ai models." Retrieved from 2304.03271 (arxiv.org)
Luccioni, Alexandra Sasha et al. (2023). "Estimating the carbon footprint of bloom, a 176b parameter language model." Retrieved from https://jmlr.org/papers/volume24/23-0069/23-0069.pdf
Patterson, David et al. (2021). "Carbon emissions and large neural network training." Retrieved from https://arxiv.org/abs/2104.10350
Wang, Peng et al. (2024). “E-waste challenges of generative artificial intelligence.” Retrieved from https://doi.org/10.1038/s43588-024-00712-6
World Economic Forum. (2024). “AI and energy: Will AI help reduce emissions or increase demand? Here's what to know.” Retrieved from https://www.weforum.org/agenda/2024/07/generative-ai-energy-emissions/
1/ Bolón-Canedo et al. (2024)
2/ Parameters are variables or values that determine how a model operates when assessing inputs and generating outputs.
3/ WEF, https://www.weforum.org/agenda/2024/07/generative-ai-energy-emissions/
4/ The training stage of development allows AI models to learn from data and to develop their abilities, while inference describes how AI applies its internal models to new data and then generates a response (Source: Oracle, https://www.oracle.com/artificial-intelligence/ai-inference/#:~:text=AI%20inference%20is%20when%20an%20AI%20model%20that%20has%20been)
5/ Zodhya, How much energy does ChatGPT consume? | by Zodhya | Medium
6/ Center for Data Innovation, https://www2.datainnovation.org/2024-ai-energy-use.pdf
7/ Lynne Kiesling, https://reason.org/commentary/data-center-electricity-use-framing-the-problem/
8/ Hugging Face, https://huggingface.co/blog/sasha/ai-environment-primer
9/ IEA, https://www.iea.org/reports/electricity-2024/executive-summary and MIT Technology Review,
https://www.technologyreview.com/2024/05/23/1092777/ai-is-an-energy-hog-this-is-what-it-means-for-climate-change/
10/ Li et al. (2023)
11/ WEF, https://www.weforum.org/agenda/2024/07/the-water-challenge-for-semiconductor-manufacturing-and-big-tech-what-needs-to-be-done/
12/ MIT Technology Review, https://www.technologyreview.com/2024/10/28/1106316/ai-e-waste/ and TechCrunch, https://techcrunch.com/2024/10/28/generative-ai-could-cause-10-billion-iphones-worth-of-e-waste-per-year-by-2030/
13/ This is the main computational component of the computer and functions analogously to the human brain.
14/ GPUs were originally designed for use in graphics cards, but it turned out that these are also better suited than CPUs to carrying out the complex matrix manipulations necessary for deep learning and the training of AI models.
15/ Luccioni et al. (2023)
16/ TPUs have been designed for use with TensorFlow, an open-source machine learning platform developed by Google that is particularly useful for deep learning projects (Source: Atichat Auppakansang, https://medium.com/super-ai-engineer/gpu-tpu-คืออะไร-ควรใช้อะไรในการ-train-model-กันแน่-1b652666cbbf)
17/ Bolón-Canedo et al. (2024)
18/ The Gradient, https://thegradient.pub/sustainable-ai/
19/ The PUE is calculated by dividing total data center energy consumption by the electricity needed to power
the computer equipment that it contains. Low values represent higher efficiencies.
20/ Cloudscene, https://cloudscene.com/region/datacenters-in-asia-pacific
21/ Google, https://research.google/blog/good-news-about-the-carbon-footprint-of-machine-learning-training/
22/ Cloud data centers hosted off-site and managed by third-party providers offer a pay-as-you-go model that can significantly reduce capital and operational expenditures. They provide greater scalability and flexibility, allowing organizations to adjust resources quickly in response to changing demands. (Source: TRG Datacenters, https://www.trgdatacenters.com/resource/cloud-data-centers-explained/)
23/ Microsoft, The Era of 1-bit LLMs: All Large Language Models are in 1.58 Bits - Microsoft Research
24/ The Register, https://www.theregister.com/2024/06/03/microsoft_spends_32b_on_expanding/
25/ Microsoft, https://natick.research.microsoft.com/
26/ Microsoft, Sustainable by design: Innovating for energy efficiency in AI, part 1 | The Microsoft Cloud Blog
27/ Amazon, https://www.aboutamazon.com/news/sustainability/amazon-renewable-energy-portfolio-january-2024-update
28/ Meta, https://sustainability.atmeta.com/2024-sustainability-report/
29/ NVIDIA, https://blogs.nvidia.com/blog/climate-research-next-wave/
30/ NVIDIA, https://www.nvidia.com/en-us/sustainability/
31/ CyberPeace, https://www.cyberpeace.org/resources/blogs/generative-ai-and-environmental-risks
32/ European Parliament, https://www.europarl.europa.eu/topics/en/article/20230601STO93804/eu-ai-act-first-regulation-on-artificial-intelligence
33/ Prior to the EU, Chile proposed a draft AI act, but this does not cover the environment.
34/ The EU AI Act divides AI risk into four levels: (i) minimal risk, e.g., video games that employ AI; (ii) specific transparency risk, e.g., requiring that AI chatbots inform users that they are interacting with a computer; (iii) high risk, e.g., medical software; and (iv) unacceptable risk, e.g., AI-powered social scoring systems (source:: European Commission, https://ec.europa.eu/commission/presscorner/detail/en/qanda_21_1683)
35/ Office of Senator Markey, https://www.markey.senate.gov/news/press-releases/markey-heinrich-eshoo-beyer-introduce-legislation-to-investigate-measure-environmental-impacts-of-artificial-intelligence
36/ Government of Canada, Government of Canada launches public consultation on artificial intelligence computing infrastructure - Canada.ca
37/ Infocomm Media Development Authority, Charting green growth for data centres in SG | IMDA
38/ Edge computing refers to a system-design concept where data is processed as close as possible to its source of origination (e.g., IoT devices, sensors, or local servers). This helps to reduce response times, and so is most suitable for systems providing some kind of real-time analysis, such as self-driving cars and virtual reality applications (source: Amity Solutions, https://www.amitysolutions.com/th/blogs/what-is-datacenter-beginners-guide)
39/ Bangkok Bank InnoHub, ทำความรู้จักเทคโนโลยี Explainable AI (XAI) ปัญญาประดิษฐ์ที่อธิบายได้ - Bangkok Bank Innohub
40/ Thansettakij, เปิดโลก Data Center ทำไม “ไทย” ดึงดูดการลงทุนจากบริษัทเทคระดับโลก (thansettakij.com)
41/ BOI, https://www.boi.go.th/index.php?page=press_releases_detail&topic_id=136139&_module=news&from_page=press_releases2
42/ AWS expects to establish AWS Asia Pacific (Bangkok) Region at the start of 2025 (source: The Standard, https://thestandard.co/aws-invest-on-datacenter-thailand/)
43/ Thansettakij, https://www.thansettakij.com/business/economy/594967
44/ Google plans to invest USD 1 billion (THB 35 billion) in a data center located on a WHA industrial estate in Chonburi and a Bangkok ‘cloud region’. This is expected to generate USD 4 billion in economic value by 2029 (source: Techsauce, https://techsauce.co/news/google-announce-investment-data-center-thailand-2024#:~:text=โดยครั้งนี้%20Google%20ได้ประกาศแผนการลงทุนกว่า%203.6)
45/ Thai PBS, https://policywatch.thaipbs.or.th/article/economy-77
46/ Prachachat, 5 เรื่องใหม่ใน PDP 2024 เขย่าโครงสร้างพลังงานฟอสซิส-หมุนเวียน
47/ Bangkok Post, https://www.bangkokpost.com/thailand/pr/2817324/pioneering-sustainable-data-centres-in-thailand
48/ Thansettakij, https://www.thansettakij.com/climatecenter/net-zero/611523