Introduction
The saying ‘data is the new currency’ has become something of a commonplace in recent years, but this reflects the core observation that within the modern business environment, data’s utility places it on a par with currency in terms of its ability to facilitate decision-making, spur innovation and build competitive advantage. Companies across the economy are thus intensifying their efforts to source and utilize data, and in turn, this is driving the development of innovative data analytics strategies as companies look to preserve and extend their competitive edge. Artificial intelligence, or AI, is one area that is attracting considerable interest, and of particular note is the ability of AI systems to determine appropriate pricing levels for goods and services. By integrating the analysis of historical data and current conditions, AI systems can accurately and rapidly evaluate supply and demand, likely consumer behavior, overall market conditions, and the factors likely to affect these. This then allows companies to set prices at their optimum level, achieve related business goals, and raise their overall levels of efficiency and productivity.
Benefits of Using AI Pricing Strategies
Artificial intelligence (AI) is an advanced technology that allows computer systems to learn from huge stores of data and to use this understanding to improve its own abilities in given areas. AI systems are generally able to produce highly accurate outputs, and in some ways, these are analogous to human cognition in their ability to assimilate, analyze and exploit information from different sources.1/ Given the promise held out by AI, many companies are now using this technology to power their pricing strategies. This involves tasking AI systems with processing extensive historical datasets alongside current information, such as the behavior of customers and trade partners, the extent of market competition and other relevant market factors. Additionally, it requires considering constraints due to the company's limitations. The goal is to conduct a multidimensional analysis that integrates these diverse elements to derive actionable insights. This then allows for the generation of an optimal price given the company’s stated aims, whether that be expanding its customer base, gaining an advantage over the opposition, achieving revenue growth, or resolving issues within the company’s own supply chains.
AI-driven pricing strategies are therefore emerging across the economy in areas as diverse as e-commerce, commercial air travel, hotels, and banking and insurance, especially in situations where companies are selling to retail customers through the internet. Being able to simultaneously adjust prices across a huge range of products gives sellers the space to gain a competitive head start and to more sharply differentiate themselves from their competitors, and so when setting prices, retailers are increasingly turning to the use of AI. Within this general field, one area that has found applications in price-setting has been machine learning (ML),2/ which allows retailers to better analyze and understand consumer behavior, overall market conditions, and other factors affecting the business environment. However, research by Fisher et al. (2023)3/ shows that analyzing historical data on only sales, prices, and product details results in an improvement in revenues or profits of at most 1%. In contrast, implementing dynamic price-response systems over a month-long testing period for 10,000 products resulted in a 15% increase in revenue and a 10% increase in profit for four pilot categories: beds, ottomans, coffee tables, and barstools.
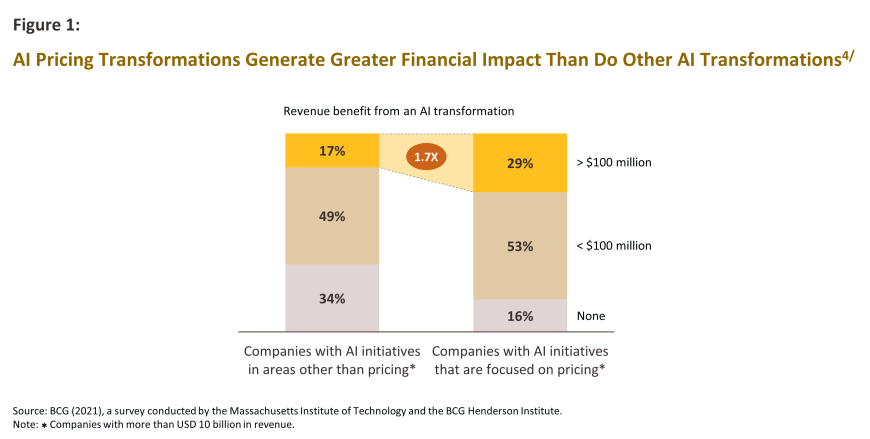
When AI processes data, it can quickly identify recurring patterns, allowing it to analyze vast amounts of information and determine optimal pricing almost instantly. This data can be internal, such as sales records, inventory data, and customer information, or external, like public data from government agencies, published research studies, and social media information. The large volume of data analyzed by AI can be categorized into two types. (i) Structured data refers to information that can be organized into a tabular format, with rows representing individual instances (e.g., a customer) and columns denoting various attributes (e.g., age, gender, income). This type of data typically originates from databases and spreadsheets and is characterized by its ease of management and processing due to its well-defined format. (ii) Unstructured data, by contrast, encompasses information that, while potentially valuable, is stored in non-repeating or irregular formats, rendering it more challenging to process. This category includes text from web forums (where users can post freely), as well as data from websites, conversations, and multimedia sources such as audio and video recordings. In addition to saving time and resources, the application of AI in data processing and analysis aids businesses in maintaining and enhancing their competitive edge, particularly in rapidly evolving industries. Specifically, the adoption of AI-driven pricing strategies often involves employing predictive technologies, such as machine learning (ML) and other statistical methods, to construct predictive models. These models are then utilized to forecast future demand and inform pricing decisions. Instead of relying on manual intervention and sequential processing, ML enables the analysis of vast datasets—commonly referred to as ‘big data’—by leveraging internal models to detect patterns, extrapolate from these patterns, and make real-time pricing decisions. Moreover, as new data and experiences accumulate, ML systems can continuously adapt, thereby improving their operational efficiency over time.
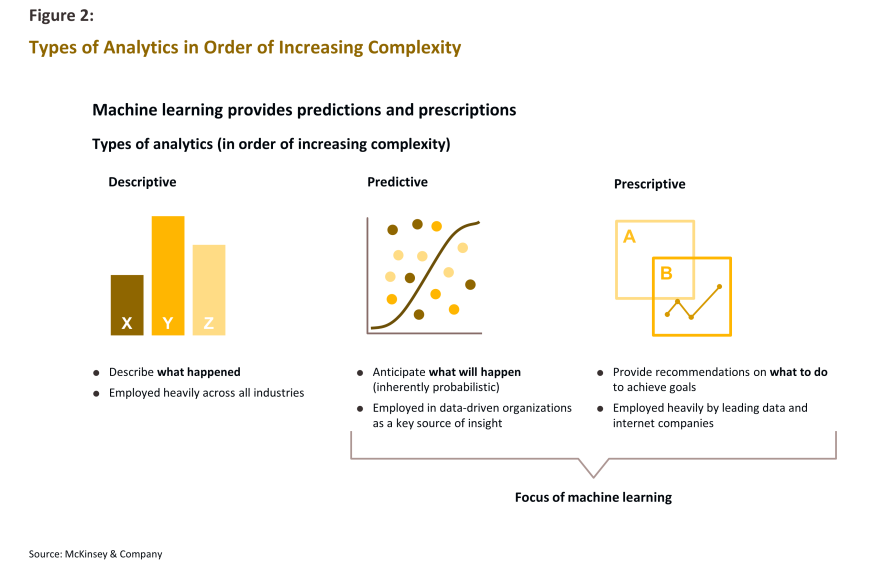
Figure 2 illustrates the role of machine learning (ML) in driving pricing strategies within the domains of predictive and prescriptive analytics. In a business-to-consumer (B2C) context, AI applications include determining prices for ride-hailing services. These systems can dynamically adjust pricing based on real-time traffic conditions and market demand, thus optimizing the balance between user/customer needs and service provider/driver requirements. Similarly, AI-driven pricing strategies can be employed in retail platforms to adjust prices in real-time when inventory is stagnant, thereby stimulating sales and restoring stock levels to desired targets. In the business-to-business (B2B) sector, manufacturers can leverage data analytics to rapidly modify the prices of goods or services in response to supply chain disruptions5/ or fluctuations in demand. Notably, research conducted by IBM in the field of commercial insurance sales indicates that the ability to offer automated quotes with a simple pricing model might lead to an increase in revenues of up to 10% when targeting small and medium-sized enterprises (SMEs).6/
AI pricing systems are capable of operating independently of human intervention, allowing them to respond promptly to rapid fluctuations in market conditions without the need for human oversight. For instance, major airlines are increasingly adopting AI-driven smart pricing systems to anticipate the responses of economy class passengers to potential upgrades to business class and to recommend optimal, individualized prices for each passenger.7/ Furthermore, research from various pilot programs suggests that the implementation of AI can significantly accelerate the selection of pricing models, reducing time-to-market8/ by 200-300% and thereby shortening the process from months to weeks.9/
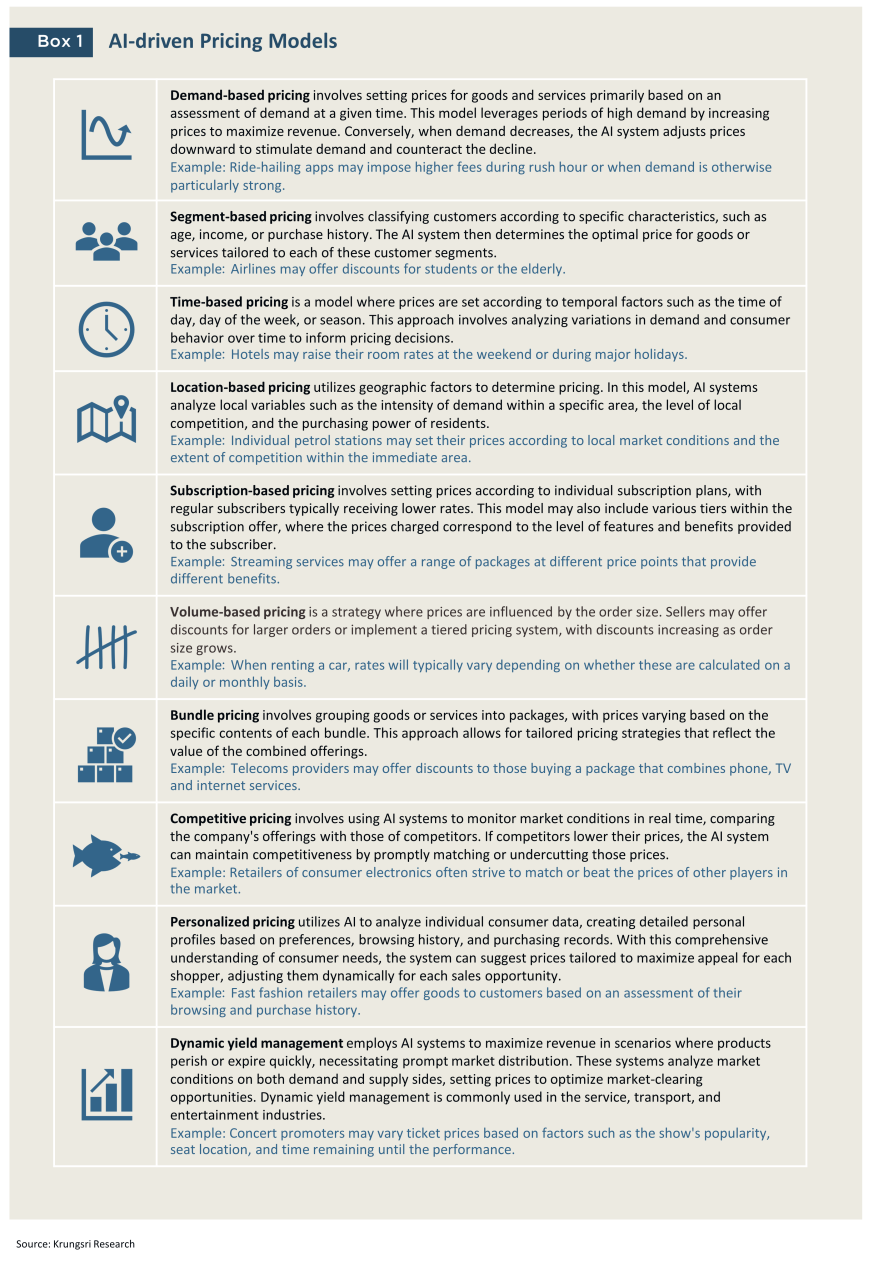
Leveraging ML to develop both predictive and prescriptive models for use in price-setting brings with it the following benefits for businesses.
-
Prices will be set at a level that best reflects current conditions. For companies in both B2B and B2C environments, combining analysis of historical data with an understanding of the prevailing market situation will allow prices to be optimized to maximally stimulate sales, thereby adding to revenues.
-
Corporate competitiveness will be boosted through AI-driven pricing strategies, enabling companies to respond swiftly to market changes or new promotions by competitors. This increased flexibility and responsiveness contribute significantly to maintaining a competitive edge.
-
AI-driven pricing enhances risk mitigation. For instance, mispricing goods and services can lead to low sales and inventory issues if prices are set too high. Furthermore, errors in forecasting market demand, whether overestimated or underestimated, can result in inappropriate pricing levels, impacting company revenue. Managing risks related to price volatility is also crucial. Effective risk management in these areas will help maintain revenues and enhance business security.
-
The quality of the customer experience will be raised through personalized pricing and tailored offerings that align with individual consumer preferences. This approach naturally leads to improved customer satisfaction and brand loyalty. Moreover, in instances where satisfaction and loyalty decline among specific target groups, and there is a heightened risk of customer attrition, strategic reductions in spending can be implemented. This includes areas such as marketing, event organization, promotions, discounts, and rewards. Making informed decisions about the likely outcomes of efforts aimed at retaining these wavering customers can thus optimize resource allocation and minimize waste in the customer retention budget.
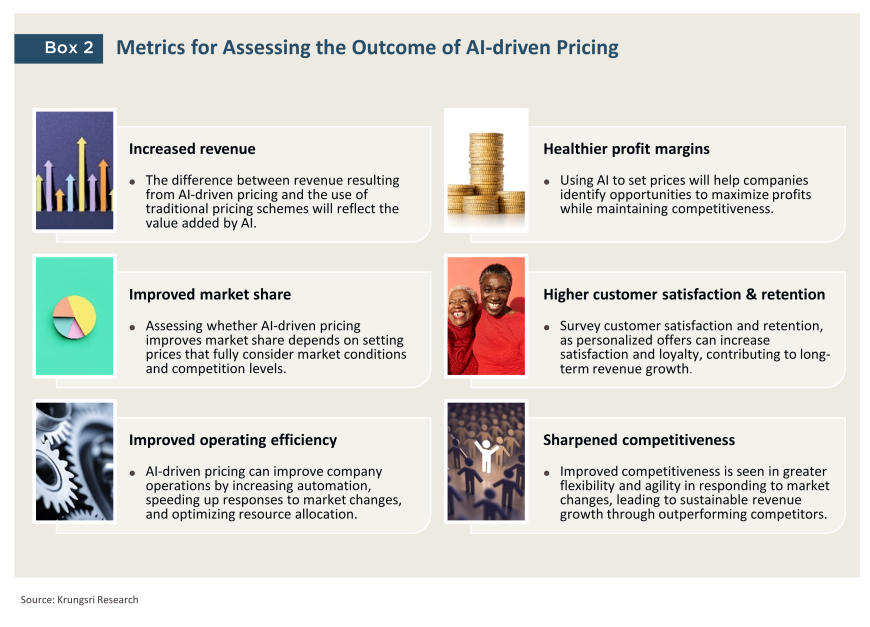
AI-driven Pricing in Financial Institutions
AI is increasingly impacting the financial sector, particularly in banking, where it enhances the efficiency of credit decision-making processes. According to McKinsey, banks are leveraging AI to automate the assessment of credit applications from retail, SME, and corporate customers, enabling near real-time decisions regarding credit issuance. This automation utilizes both structured and unstructured data from a variety of traditional sources, such as banking transactions, credit histories, and tax returns.10/ Additionally, alternative data sources, including business locations, telecom network usage, and utility bills, are incorporated to support these evaluations.11/ This integration of diverse data sources allows for more comprehensive risk assessments and streamlined decision-making processes.
Banks can enhance credit decision-making by employing machine learning (ML) models and advanced analytics across diverse datasets. This approach enables rapid assessment, increasing lenders' confidence in evaluating borrowers' creditworthiness, and facilitates the setting of appropriate credit limits, loan amounts, and interest rates. ML also mitigates fraud by improving the detection of anomalies, such as falsified loan submissions or irregular credit card transactions. Real-time monitoring of credit card spending allows comparison with historical consumer behavior, and significant deviations from these patterns may indicate potential fraudulent activity.
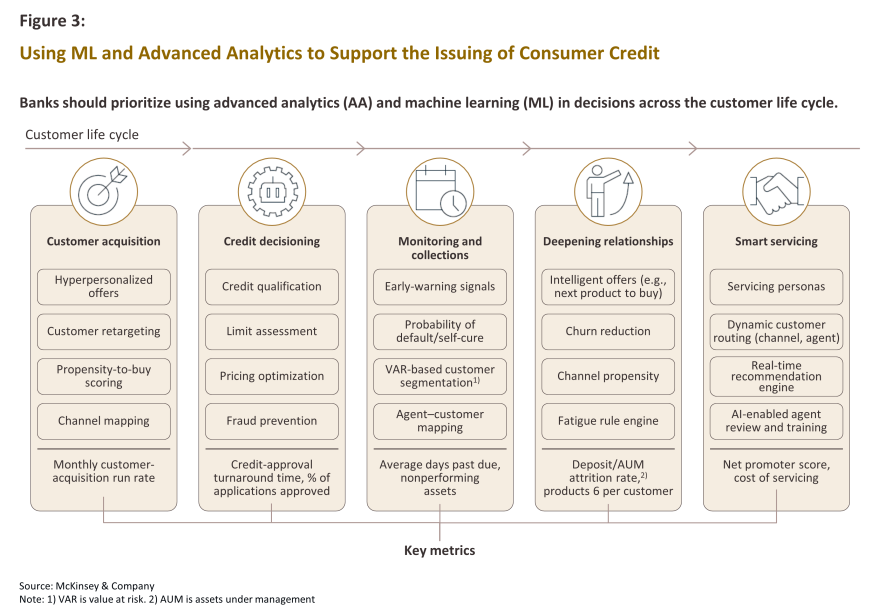
In addition, AI systems enhance financial institutions' ability to rapidly and effectively analyze big data and other relevant factors. This capability facilitates more accurate pricing of financial products and services for individual customers and consumer segments. Such precision is essential in high-risk markets, such as credit and loans, where machine learning can be applied to risk scoring and loan pricing. As a result, forward-thinking banks can offer loans at rates that are both attractive to borrowers and aligned with the bank's risk exposure, while minimizing associated costs.
In Thailand, the implementation of risk-based pricing (RBP), which involves adjusting interest rates based on the risk profile of individual borrowers, is currently under investigation through the Bank of Thailand’s RBP sandbox. This sandbox permits financial institutions with developed risk-assessment systems or models to apply these tools to determine interest rates for loans issued within the sandbox framework. The objective is to gain insights into the efficacy of these risk assessment mechanisms, especially for higher-risk borrowers. Financial institutions participating in this initiative must still adhere to the Bank of Thailand’s regulations regarding the issuance of personal loans under supervision and/or nano finance under supervision, as well as the broader principles of responsible lending. However, these institutions are allowed to exceed the current interest rate ceiling, enabling them to charge interest, penalties, fees, and other charges beyond existing limits. This flexibility aims to integrate high-risk borrowers more comprehensively into the formal banking sector. Conversely, low-risk borrowers or those with a strong repayment history may benefit from lower-cost financing, potentially encouraging a culture of responsible borrowing. Looking ahead, banks leveraging innovative AI applications could extend these advancements to address financing accessibility challenges faced by certain segments of society and tackle the high levels of household debt prevalent in the country.
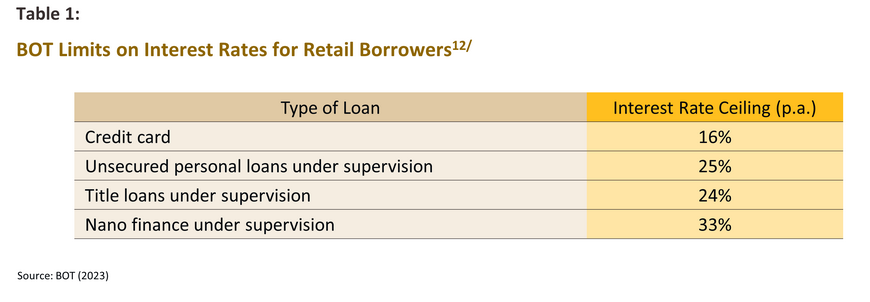
Currently, a substantial number of banks are employing AI systems to set prices for financial services and products. Applications of this technology include providing relationship managers (RMs) with suggested pricing strategies when pitching loan products to clients, offering personalized microloans with customized terms and conditions for retail borrowers, and establishing pricing models to mitigate customer churn rates. According to EY Global,13/ some banks leverage AI to determine optimal deal prices for relationship managers to use during negotiations with clients. These AI-driven decision support systems are designed specifically to furnish relationship managers with highly targeted, real-time data and analysis pertinent to their immediate context. This information is delivered via a smart advisor application that consolidates historical data and integrates it with formalized systems that encapsulate the bank’s expertise in structuring commercial deals. Consequently, relationship managers can present clients with the most advantageous proposals within a significantly reduced timeframe. This not only enhances operational efficiency and productivity but also allows bank staff to use the saved time to strengthen customer relationships and engage in other tasks.
Interest in AI-driven pricing extends to neobanks or virtual banks as well. In January 2022, the major Brazilian virtual bank Nubank acquired the US-based AI startup Olivia. This acquisition has significantly enhanced Nubank's capacity to leverage AI in developing new financial products and refining operating procedures and customer service. Nubank now utilizes AI to offer personalized microloans based on an analysis of individual customer data, including spending patterns, utility bill payments, and online and social media activity.14/ This data is used to evaluate customers' creditworthiness and determine loan terms and prices tailored to each individual’s specific circumstances. Nubank’s competitive advantage lies in its ability to penetrate retail borrower markets and set optimal pricing for each loan. This approach has proven successful, allowing Nubank to offer financial products via its mobile app at more competitive rates (in regard to fees and/or interest) compared to its rivals. As a result, Nubank attracted nearly 20 million new customers within a single year. By the end of 2023, the bank, which has been operational for only a decade, had accumulated a total of 93.9 million customers across Brazil, Mexico, and Colombia.15/
Another notable advantage of employing AI to determine individualized pricing and conditions for borrowers is its capacity to enhance banks' responsiveness to consumer needs, thereby significantly improving the overall customer experience and fostering greater loyalty. Research by Zarkesh (2023), which examines customer churn rates and loyalty indicators in the market for health insurance sold by Iranian banks, indicates that traditional non-AI-enabled pricing systems result in approximately 30% of customers not renewing their insurance upon expiration. In contrast, the introduction of AI-driven pricing strategies reduced the customer churn rate to 20% (see Figure 4). This evidence suggests that AI-driven pricing can substantially contribute to customer retention. Furthermore, since retaining existing customers is typically more cost-effective than acquiring new ones, the use of AI-driven pricing strategies also has the potential to reduce operating expenses.
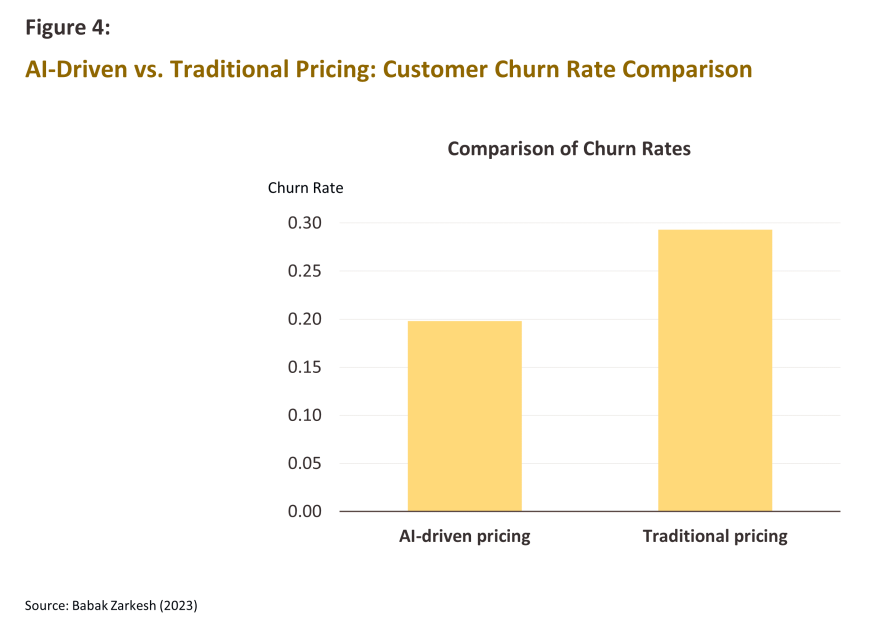
Nevertheless, it is important to note that the banking and finance industry differs in a significant regard from other industries in that banks are not able to adjust fees and interest rates on a real-time basis. This puts banking in a different position to, for example, airlines, which can adjust the prices advertised on their website by the second, or online retail platforms, which can slash their prices during sales promotions. By contrast, financial products are much more tightly regulated, and this poses a considerable challenge for banks when attempting to determine optimum pricing strategies. Additionally, banks often encounter data shortfalls, making it difficult to gather comprehensive information required for an in-depth analysis of customer needs and profiles. Distinguishing between internal and external factors that drive changes in customer behavior—such as economic cycles, social shifts, or environmental influences—presents another challenge. Furthermore, banks must navigate the complexities of managing risk across various dimensions. Adjustments in interest rates and loan conditions have direct implications for the institution's exposure to credit-related risk and the adequacy of its capital reserves.
Krungsri Research view
Implementing AI-driven pricing strategies has the potential to generate a wide range of positive outcomes, including addressing consumer needs, promoting business growth, and supporting innovation, which collectively drive overall economic development. For consumers, pricing that aligns with their willingness to pay enhances satisfaction by meeting both their needs and purchasing power. Setting prices in accordance with individual purchasing power allows consumers to freely choose the goods and services they require, thereby increasing their utility, as per economic theory.
For companies, the ability to accurately determine optimal pricing levels through AI can enhance competitiveness across varying market conditions. AI's capacity to analyze a vast array of demand- and supply-side factors in real time enables the implementation of pricing strategies that can boost sales and revenue compared to non-AI-enabled conditions, particularly in bullish and expansionary markets. Ideally, AI-driven pricing will achieve an optimal balance between fulfilling consumer demand and managing inventory efficiently. For instance, it can facilitate the accelerated sale of perishable or time-sensitive goods and assist in clearing out old stock in preparation for new inventory. Conversely, in bearish and contractionary markets—such as those characterized by supply chain disruptions or reduced consumer confidence—AI can offer pricing recommendations that help clear the market while maintaining stability. This involves balancing the need to maximize company revenues with setting prices that reflect consumers' constrained purchasing power. If companies can resist the temptation to fully pass increased costs onto customers, they will continue to sell products and maintain business viability. Therefore, AI-driven pricing not only benefits the business by enhancing revenue and competitiveness but also supports consumers and contributes positively to the broader economy.
However, it is important to remember that when moving into this area, companies cannot afford to overlook issues relating to corporate transparency, and it is crucial that businesses communicate clearly when setting and adjusting prices. This includes how customers or trade partners will benefit from the use of AI-enabled pricing strategies. Being diligent in this area will then help to build trust on both sides of the market, reduce the risk of reputational damage, assure consumers that the company is fully committed to its customers and will remain responsive to them, and underscore the business’s commitment to fair practices and customer-centric operations.
Businesses will also need to pay extremely close attention to issues connected to privacy and how this relates to their usage and storage of customer data to foster customer confidence. Because AI relies on huge datasets for its training and operations, companies will need to make extended efforts to ensure that customer data remains both private and safe. This will clearly involve meeting the regulatory requirements for secure data storage, but beyond this, companies will need to communicate with their customers and to assure these that the business is and will always remain a reliable custodian of their data.
Finally, to fully comprehend the impacts of AI-enabled pricing on all stakeholders, businesses must remain receptive to and critically evaluate any feedback they receive and be prepared to act upon this information as necessary. For example, in 2024, Wendy’s, a fast-food chain operating over 7,000 restaurants in the United States, announced its intention to implement AI-powered dynamic pricing, which included the integration of AI-driven adjustments to menu prices. This decision was widely perceived as an effort to maximize revenue by increasing prices during peak periods, raising concerns among commentators that such a strategy would disproportionately affect low-income groups, who rely heavily on affordable fast-food options. In response to this backlash, Wendy’s clarified that the intent behind the AI implementation was to enhance value through additional offers and discounts, rather than solely to raise prices.16/ This example underscores the importance of transparent communication and responsiveness to public perception in the successful adoption of AI-driven pricing strategies.
Returning to the Thai banking and finance sector, as stated above, although real-time management of loan fees and interest rates through AI remains unfeasible, AI continues to play a significant role in both front- and back-office operations. Applications of AI include addressing staff and customer inquiries, optimizing internal processes, assessing credit risk, and determining appropriate loan interest rates, thereby supporting the Bank of Thailand’s transition towards risk-based pricing (RBP) measures. Furthermore, starting in 2025, the Bank of Thailand will commence issuing licenses for virtual banks, with one major criterion being the prospective bank’s ability to introduce innovative products that are distinct from competitors, effectively meet consumer needs, and manage costs. In this context, AI-driven pricing could serve as a potential selling point or a key differentiator for applicants seeking virtual bank licenses, akin to the approach taken by Nubank.
It is evident that, even at present, AI systems offer substantial capabilities, and ongoing advancements in the field suggest that these capabilities will continue to expand in the future. The technology will thus create an increasingly broad range of use cases for businesses generally, and particularly within the banking sector. In this rapidly evolving landscape, a significant challenge for companies will be to identify strategies to optimize the potential of AI. This involves striking an ideal balance between addressing consumer needs and generating revenue, while adhering to ethical business practices and complying with all regulatory and legal requirements. Successfully navigating these challenges will result in substantial benefits for consumers, businesses, and the economy overall.
References
Akshat Agarwal, Charu Singhal, and Renny Thomas (2021) AI-powered decision making for the bank of the future, McKinsey & Company. Retrieved March 12, 2024 from https://www.mckinsey.com/industries/financial-services/our-insights/ai-powered-decision-making-for-the-bank-of-the-future
Babak Zarkesh (2023) Exploring the Impact of AI-Driven Pricing on Customer Loyalty and Churn Rates in the Banking Industry (Master’s thesis), Norwegian University of Science and Technology (NTNU) Faculty of Economics and Management. Retrieved April 17, 2024 from https://ntnuopen.ntnu.no/ntnu-xmlui/handle/11250/3080341
BOT’s Consultation Paper (2023) (ร่าง) กลไกการกำหนดอัตราดอกเบี้ยตามความเสี่ยงสำหรับสินเชื่อรายย่อย (Risk-based pricing) และการทดสอบใน Sandbox, ฝ่ายนโยบายกำกับสถาบันการเงิน สายนโยบายสถาบันการเงิน, September 2023. Retrieved May 19, 2024 from https://www.bot.or.th/content/dam/bot/documents/th/laws-and-rules/hearing/public-hearing-202309-rbp.pdf
Joël Hazan et al. (2021) Why AI Transformations Should Start with Pricing, BCG publication, June 2021. Retrieved May 19, 2024 from https://www.bcg.com/publications/2021/ai-pricing-tranformations
Josephine Maltby (2016) Should all politicians publish their tax returns? Here’s what we might find, The Conversation, Retrieved June 25, 2024 from https://theconversation.com/should-all-politicians-publish-their-tax-returns-heres-what-we-might-find-57678
Koos Quak and Gert-Jan van Zuijlen (2019) Dynamic pricing with AI syncs insurers with market realities, Expert Insights, IBM Institute for Business Value, August 2019. Retrieved March 12, 2024 from https://www.the-digital-insurer.com/wp-content/uploads/2020/05/1578-74027274usen-02_74027274USEN.pdf
Marshall Fisher, Santiago Gallino, and Jun Li (2023) A Step-by-Step Guide to Real-Time Pricing, Harvard Business Review, November-December 2023. Retrieved April 17, 2024 from https://hbr.org/2023/11/a-step-by-step-guide-to-real-time-pricing
Matt Cox et al (2023) Using AI to augment pricing intelligence for banks, EY Global - Banking and Capital Markets, December 2023. Retrieved May 19, 2024 from https://www.ey.com/en_gl/insights/banking-capital-markets/using-ai-to-augment-pricing-intelligence-for-banks
McKinsey Analytics (2018) An executive’s guide to AI, McKinsey & Company. Retrieved April 17, 2024 from https://www.mckinsey.com/~/media/McKinsey/Business%20Functions/McKinsey%20Analytics/Our%20Insights/An%20executives%20guide%20to%20AI/An-executives-guide-to-AI.ashx
Nasser Sami Zagha (2024) Artificial Intelligence Real Use Cases for Credit scoring in Banks and Fintech firms, February 2024. Retrieved April 17, 2024 from https://www.linkedin.com/pulse/artificial-intelligence-real-use-cases-credit-scoring-nasser-sami-ee6be
Nu Holdings Ltd. Reports Fourth Quarter and Full Year 2023 Financial Results. Retrieved April 17, 2024 from https://international.nubank.com.br/company/nu-holdings-ltd-reports-fourth-quarter-and-full-year-2023-financial-results
Shelley E. Kohan (2024), Wendy’s Dynamic Pricing Announcement Puts New CEO Kirk Tanner In Spotlight, Forbes. Retrieved April 17, 2024 from https://www.forbes.com/sites/shelleykohan/2024/03/02/wendys-dynamic-pricing-announcement-puts-new-ceo-kirk-tanner-in-spotlight/?sh=6c39110b72d2
1/ https://www.krungsri.com/th/plearn-plearn/credit-card-ai-generation
2/ Machine Learning (ML) is an application of AI that uses data and mathematical models to enable computers to learn automatically without being explicitly programmed. This allows computer systems to learn, develop, and improve themselves based on experience. In addition to ML, other AI subfields include Deep Learning, Robotics, Neural Networks, Natural Language Processing (NLP), and Genetic Algorithms. Each of these fields plays a crucial role in shaping the future of AI and has the potential to revolutionize various industries worldwide.
3/ https://hbr.org/2023/11/a-step-by-step-guide-to-real-time-pricing
4/ https://www.bcg.com/publications/2021/ai-pricing-tranformations
5/ Supply chains can be disrupted by a huge number of factors. Natural disasters (e.g., earthquakes or hurricanes) can cause damage to infrastructure and impact communications routes, problems with suppliers (e.g., production delays or bankruptcies) can delay the delivery of goods, and logistics problems (e.g., portside congestion and slow customs clearance) can hinder the movement of products. In addition, political and economic factors can also negatively impact supply chains. Thus, trade disputes and volatility in currency markets may drag on supply chains globally, labor shortages or disputes may disrupt production lines, and problems with technology (e.g., equipment malfunctions and software failures) can affect operations. Moreover, as the Covid-19 experience showed, outbreaks of disease and other types of public health crisis can also have widespread impacts on supply chains.
6/ IBM Institute for Business Value analysis, based on unpublished project data from "Dynamic Pricing with AI Syncs Insurers with Market Realities."
7/ IBM Institute for Business Value analysis, based on secondary research from "Dynamic Pricing with AI Syncs Insurers with Market Realities."
8/ Time to Market (TTM) refers to the total duration required from the initiation of a product or service concept to its successful launch in the market. TTM is a critical business metric because, when products are similar, a company with a shorter TTM can capitalize on new market opportunities more quickly than its competitors, thus gaining a competitive advantage.
9/ IBM Institute for Business Value analysis, based on unpublished project data from "Dynamic Pricing with AI Syncs Insurers with Market Realities."
10/ In Thailand, tax return information for individuals is not publicly disclosed. However, in some countries, such as Norway, this data is accessible online. Retrieved June 25, 2024 from https://theconversation.com/should-all-politicians-publish-their-tax-returns-heres-what-we-might-find-57678
11/ Note that access to new, alternative sources of data is dependent on progress on ‘open banking’, trends in sharing other information, the existence of APIs that make data available to 3rd parties, and the official granting of permission to data aggregators to operate in particular markets. Moreover, it is imperative that when accessing and using personal information, banks work tirelessly to maintain data security and to protect customer privacy, as laid out in the relevant regulatory framework, e.g., the PDPA.
12/ https://www.bot.or.th/content/dam/bot/documents/th/laws-and-rules/hearing/public-hearing-202309-rbp.pdf
13/ https://www.ey.com/en_gl/insights/banking-capital-markets/using-ai-to-augment-pricing-intelligence-for-banks
14/ https://www.linkedin.com/pulse/artificial-intelligence-real-use-cases-credit-scoring-nasser-sami-ee6be, Retrieved April 17, 2024.
15/ https://international.nubank.com.br/company/nu-holdings-ltd-reports-fourth-quarter-and-full-year-2023-financial-results/, Retrieved April 17, 2024.
16/ https://www.forbes.com/sites/shelleykohan/2024/03/02/wendys-dynamic-pricing-announcement-puts-new-ceo-kirk-tanner-in-spotlight/?sh=6c39110b72d2